Predictions For Big Data Analytics And Cognitive Computing In 2016
- Gil Press, Adrian Bridgwater
- Jan 17, 2016
- 6 min read
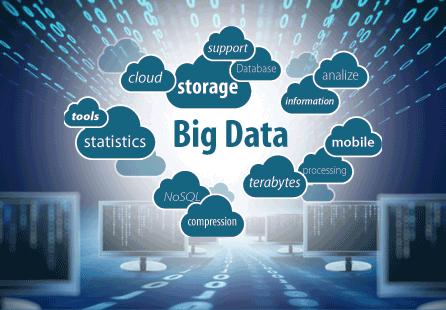
Big data analytics is the process of examining big data to uncover hidden patterns, unknown correlations and other useful information that can be used to make better decisions.
The demand for enterprise professionals with data analytics skills continues to grow, as does the competition for top analytics talent. Data analytics, though, comes in many flavors and derivations, which means some specialties and skill sets are more in demand than others.
Data analytics platform and services vendor CrowdFlower just conducted an interesting analysis of LinkedIn job postings for data analytics professionals. The company reviewed about 3,500 relevant job openings from the online networking site for professionals.
Four key elements of big data analytics Named (most probably) after its founders Ross Ihaka and Robert Gentleman, R performs at its best when used for big data statistics, predictive modeling and machine learning. In terms of use, we must now appreciate that there is more than one type of analytics ‘thing’ or ‘function’ that we might want to do: 1. Big data analytics can be data preparation — elements such as de-duplication and time stamping data to know when it was created. 2. Big data analytics can be data exploration — finding out what the core characteristics of the data set are. 3. Big data analytics can be data visualization — charts and graphs to make interpreting data trends easier. 4. Big data analytics can be data modeling — building up the logic so we know how different parts of data relate to each other.

Enough big data foundations already, what Microsoft is doing here is making sure that R Server boasts really good multiplatform support and is essentially open from the core. Remember how Microsoft has (arguably impressively) flummoxed us all by getting the open source religion and preaching it from every minaret in town? This, in effect, is a play for one big data tool to rule them all if you will.
ocating more of their budget dollars toward analytics. After all, if they don’t, their competitors will.
Big data analytics is the next trillion-dollar market, says Michael Dell. IDC has a more modest and specific prediction, forecasting the market for big data technology and services to grow at a 23.1% compound annual growth rate, reaching $48.6 billion in 2019.
The larger market for business analytics software and business intelligence solutions which now includes the new disciplines of data science and cognitive computing (e.g., IBM IBM -1.54%’s Watson) is at least 5 times bigger. But a much larger market, which may indeed approach a trillion dollar sometime in the not-distance future, includes the revenues companies in any industry will generate from “monetizing” their data and algorithms.
Here’s my summary of predictions for big data analytics and cognitive computing from the International Institute for Analytics (IIA), Forrester, IDC, and Gartner IT +1.16%.
Big data analytics will be embedded everywhere
IIA predicts that computing will become increasingly microservice-enabled, where everything – including analytics – will be connected via an API. IDC predicts that by 2020, 50% of all business analytics software will include prescriptive analytics built on cognitive computing functionality and that Cognitive Services will be embedded in new apps. Embedded data analytics will provide U.S. enterprises $60+ billion in annual savings by 2020.
IDC analyst for business analytics and information management Dan Vesset is convinced that Microsoft is playing an ‘important role’ (his words) in bringing big data analytics modeling and productivity tools and deployment tools to a broader audience.
Is Microsoft doing well in open source big data analytics? Would that there was a highly amusing sarcastic remark to make as an epitaph here… almost none of the trade press were scathing to any degree whatsoever and one even used ‘Hooray!’ in the headline. It’s all about market domination though isn’t it? Microsoft is no charity. That’s that about as caustic as we can get here.
Data Security
The year 2015 was one of escalating breaches for banking, healthcare, government, media and telecommunications. No industry sector was spared, and these attacks demonstrated their destructive capabilities. Nation-state activity increased to an all-time high, paving the road for the cybersecurity pact with China. From a technology point of view, social, mobile, big data, and cloud transitioned from buzzwords to the new normal. In 2016, I expect cyberthreats will continue to increase. Whether or not the cybersecurity pact
leads to a framework of new international norms remains to be seen. Cloud continues to mature and will see adoption by large companies that only a year or two ago would have never considered it as an option.
In 2016, cloud will be about leveraging new capabilities rather than just a cost savings. Analytics and cognitive capabilities will see rapid growth as organizations look at their big data for new insights.
Mobile Mobile applications must be fast, responsive, and reliable to meet end users’ demanding expectations. To meet these demands, mobile developers are no longer building applications for the best-case performance scenario; they are building apps for the worst-case scenario – guaranteeing an ideal mobile experience regardless if connectivity is ideal or spotty. To stay competitive, companies will take an offline-first mobile development approach so business performance is no longer tied to uncontrollable network performance. – Bob Wiederhold, CEO, Couchbase
Data Management Next-generation, software-based storage technology is enabling multi-temperature (fast and dense) solutions. Flash memory is a key technology that will enable new design for products in the consumer, computer, and enterprise markets. Consumer demand for flash will continue to drive down its cost, and flash deployments in big data will begin to deploy. The optimal solution will combine flash and disk to support both fast and dense configurations. In 2016, this new generation of software-based storage that enables multi-temperature solutions will proliferate so organizations will not have to choose between fast and dense – they will be able to get both. – John Schroeder, CEO and co-founder, MapR
Open Source MapReduce is quite esoteric. Its slow batch nature and high level of complexity can make it unattractive for many enterprises. Spark, because of its speed, is much more natural, mathematical, and convenient for programmers. Spark will reinvigorate Hadoop and, in 2016, nine out of every 10 projects on Hadoop will be Spark-related projects. – Monte Zweben, co-founder and CEO of Splice Machine and executive chairman of RocketFuel
Machine Learning In 2016, every company will want to get on the machine-learning bandwagon. But without the right people, many won’t have the expertise to do it. Expect to see the development of turnkey databases that allow developers to build predictive models without having a Ph.D. – Monte Zweben, co-founder and CEO of Splice Machine and executive chairman of RocketFuel
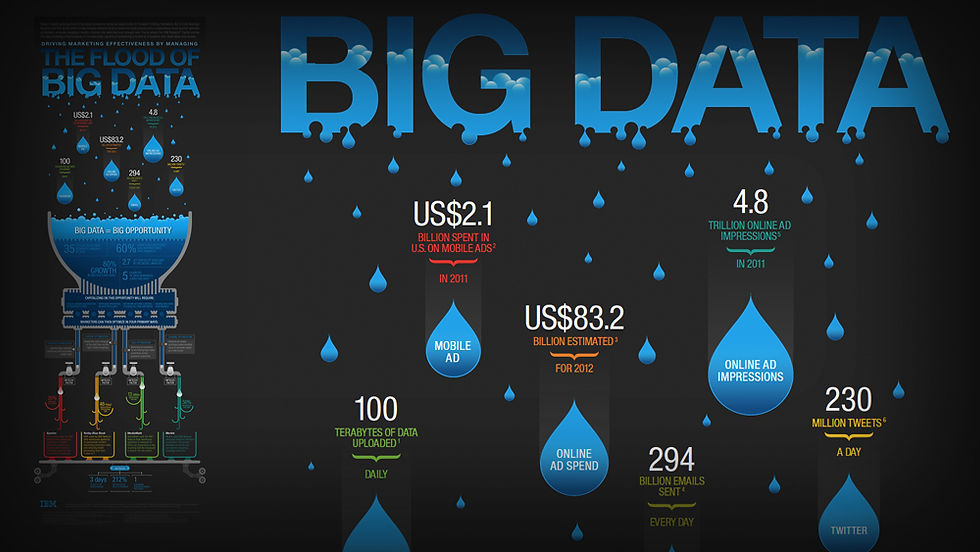
Reactive vs. proactive approaches Reactive – business intelligence. In the reactive category, business intelligence (BI) provides standard business reports, ad hoc reports, OLAP and even alerts and notifications based on analytics. This ad hoc analysis looks at the static past, which has its purpose in a limited number of situations. Reactive – big data BI. When reporting pulls from huge data sets, we can say this is performing big data BI. But decisions based on these two methods are still reactionary. Proactive – big analytics. Making forward-looking, proactive decisions requires proactive big analytics like optimization, predictive modeling, text mining, forecasting and statistical analysis. They allow you to identify trends, spot weaknesses or determine conditions for making decisions about the future. But although it's proactive, big analytics cannot be performed on big data because traditional storage environments and processing times cannot keep up. Proactive – big data analytics. By using big data analytics you can extract only the relevant information from terabytes, petabytes and exabytes, and analyze it to transform your business decisions for the future. Becoming proactive with big data analytics isn't a one-time endeavor; it is more of a culture change – a new way of gaining ground by freeing your analysts and decision makers to meet the future with sound knowledge and insight.
Goodbye data preparation, hello data science
IIA predicts that automated data curation and management will free up analysts and data scientists to do more of the work they want to do. Forrester says that in 2016, machine learning will begin to replace manual data wrangling and data governance dirty work, and vendors will market these solutions as a way to make data ingestion, preparation, and discovery quicker. Through 2020, according to IDC, spending on self-service visual discovery and data preparation tools will grow 2.5x faster than traditional IT-controlled tools for similar functionality.
One tool to rule them all
Comments